Deep Learning Demystified: From Jigsaw Puzzles to AI Breakthroughs
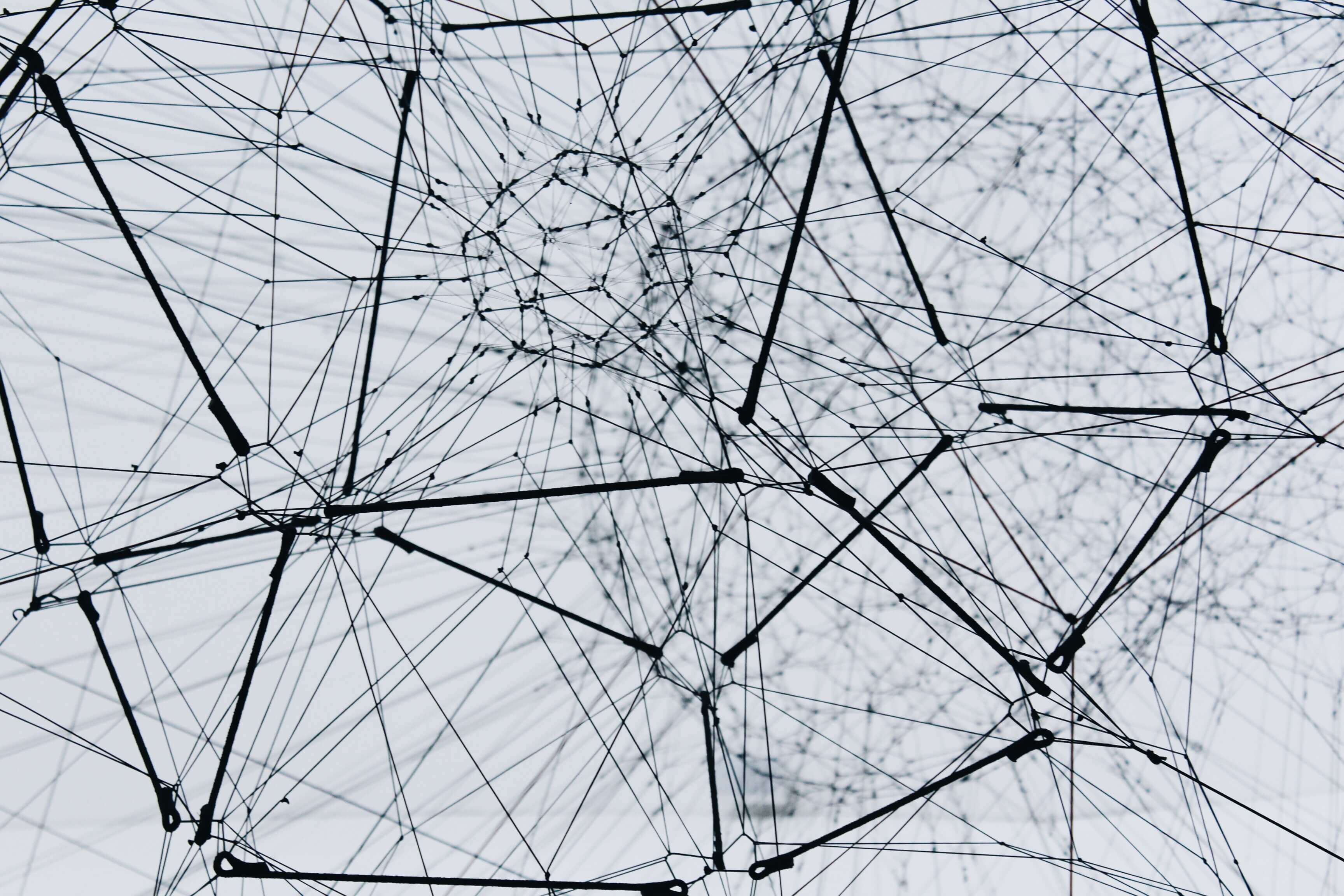
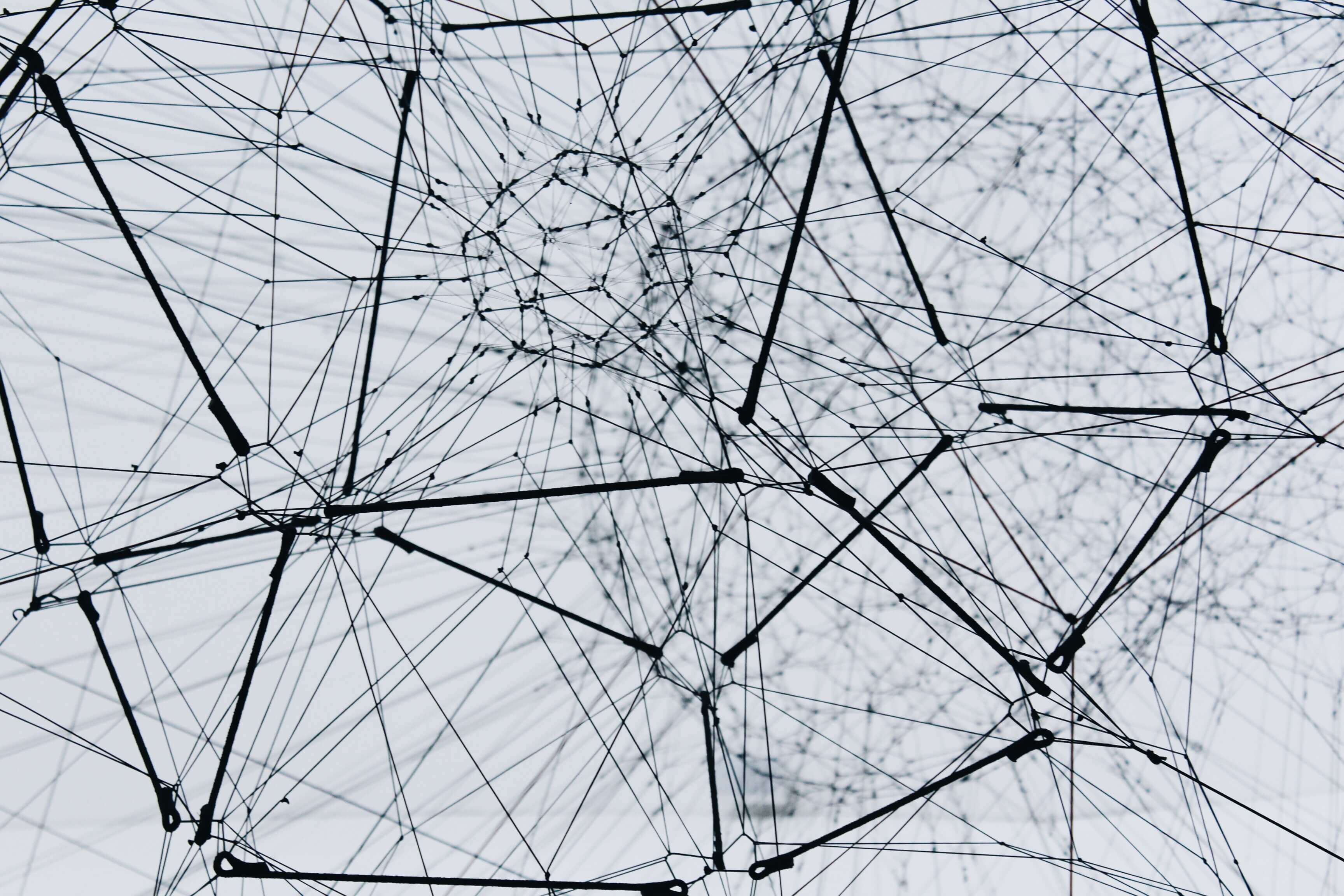
Many of the most recognizable applications of AI are due to recent advancements in a field of Machine Learning called: Deep Learning. Personal assistants like Google, Amazon Alexa, and Siri are examples of AI that involve this area of ML. Although these personal assistants are prone to error, their accuracy and usefulness have greatly improved over the past few years. With more data generated than ever, and computing power continuing to improve, Deep Learning algorithms can better classify images, recognize faces, and make increasingly accurate predictions.
To conceptualize Deep Learning, think of building a jigsaw puzzle. The builder dumps the pieces onto a table and begins to sort. First, they start with the border pieces; picking out all of these pieces and beginning to build the perimeter of the puzzle. Once this is complete, they may start identifying pieces that have similar colors or patterns and group them together. Using the information from the borders, the image begins to take shape.
Deep Learning, like building a jigsaw puzzle, contains several layers. Each layer helps to break down part of the problem and contributes to identifying the solution. Each of the layers are called neurons and groups of layers are called neural networks. The name Deep Learning refers to the fact that these neural networks often contain several layers or ‘steps’ to solve the problem.
When a neural network identifies an image- for example an image of the letter ‘A’- it receives this image as a collection of pixels. Pixels are tiny 1x1 squares that together make up the overall image. The algorithm receives these pixels in the form of 3 numbers. This combination of numbers represents the color in a given pixel. In one layer of the neural network, the Deep Learning algorithm is looking for combinations of pixels and trying to identify the edges of the image. The next layer may look for different colors or saturation within combinations of pixels. The algorithm then determines what the image ‘looks’ like and compares the identified image with those that have been previously identified as the letter ‘A’ (it compares using images the algorithm has been trained on). Neural Networks require a large number of examples to successfully learn how to identify images; this is why the increase of data availability is so important to the successful application of Deep Learning.
Deep Learning was invented in 1965; with Canadian Geoffry Hinton making important contributions in 1986. Although the mathematical and algorithmic approaches to Deep Learning were discovered 40+ years ago, a lack of computing power and data availability limited their use. Computing power has doubled every two years since Deep Learning was invented and the introduction of digital technologies has resulted in an overwhelming amount of data collection. With these advancements, Deep Learning has made a comeback and is rapidly improving the abilities (such as seeing and communicating) we’ve come to expect from AI.
Some of the success of Deep Learning is apparent in the reduction of error rates in image recognition by 41%, facial recognition by 27%, and voice recognition by 25%. As error rates are reduced companies have begun to apply the technology and have made it available for everyday use. Smart speakers (like Amazon Echo), Google Translate, and unlocking your phone just by looking at the camera, are all examples of Deep Learning in action. The largest technology companies have been some of the first to harness the power of Deep Learning because of their ability to collect data from consumers. Every time an email is sent in Gmail, an address is entered in Apple Maps, or a purchase is made on Amazon, data is being collected and fed into these neural networks.
Some other applications of Deep Learning include:
- Diagnosing diseases from medical scans
- Detection of defective products on assembly line
- Identification of fraudulent credit card transactions
Companies who take advantage of these recent developments and allow Deep Learning to put the puzzle pieces together, can greatly improve predictive accuracy and free up human resources to provide better customer experiences.
Chapters

Mitchell Johnstone
Director of Strategy
Mitch is a Strategic AI leader with 7+ years of transforming businesses through high-impact AI/ML projects. He combines deep technical acumen with business strategy, exemplified in roles spanning AI product management to entrepreneurial ventures. His portfolio includes proven success in driving product development, leading cross-functional teams, and navigating complex enterprise software landscapes.