Understanding Data Biases in Machine Learning: Lessons from Politics
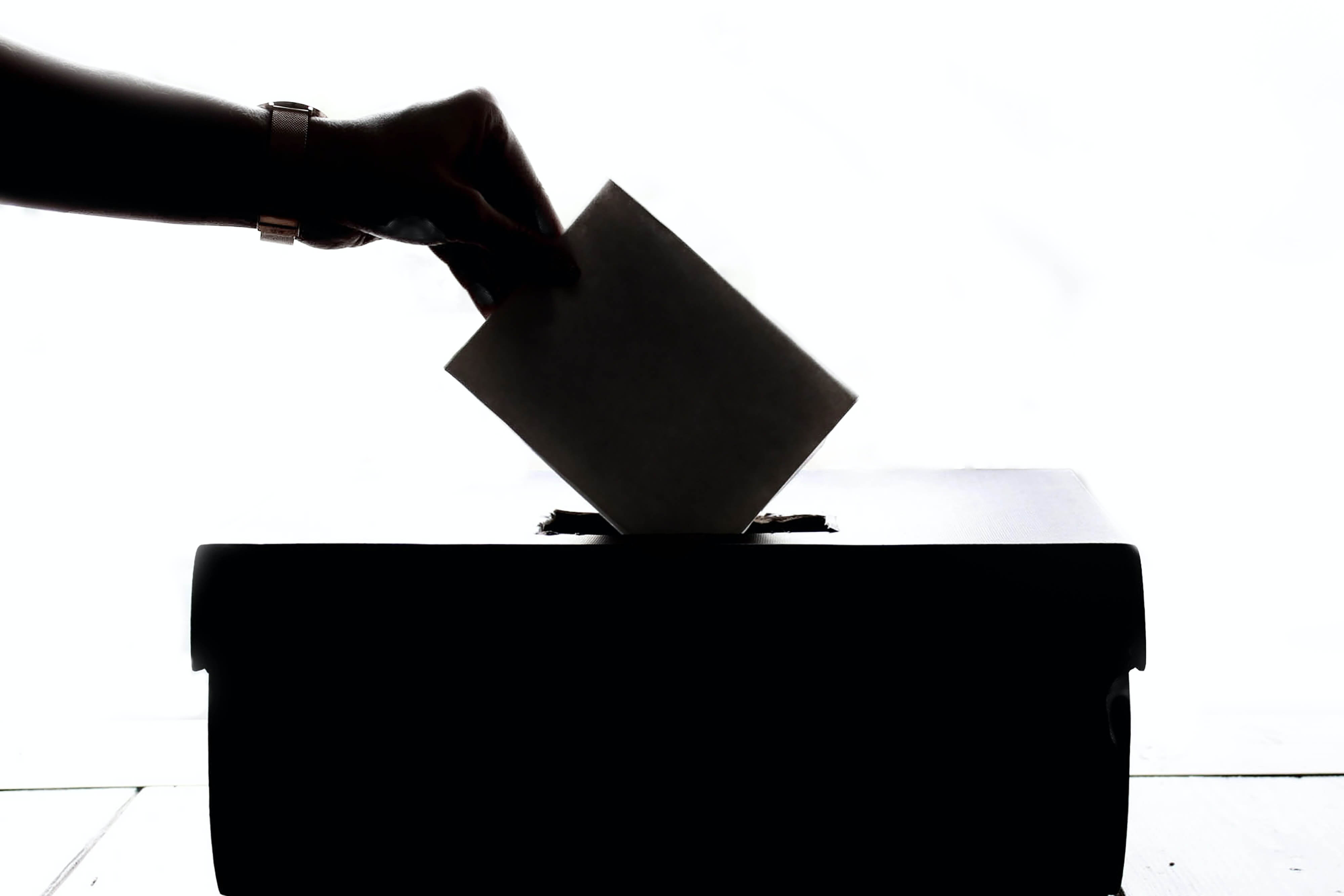
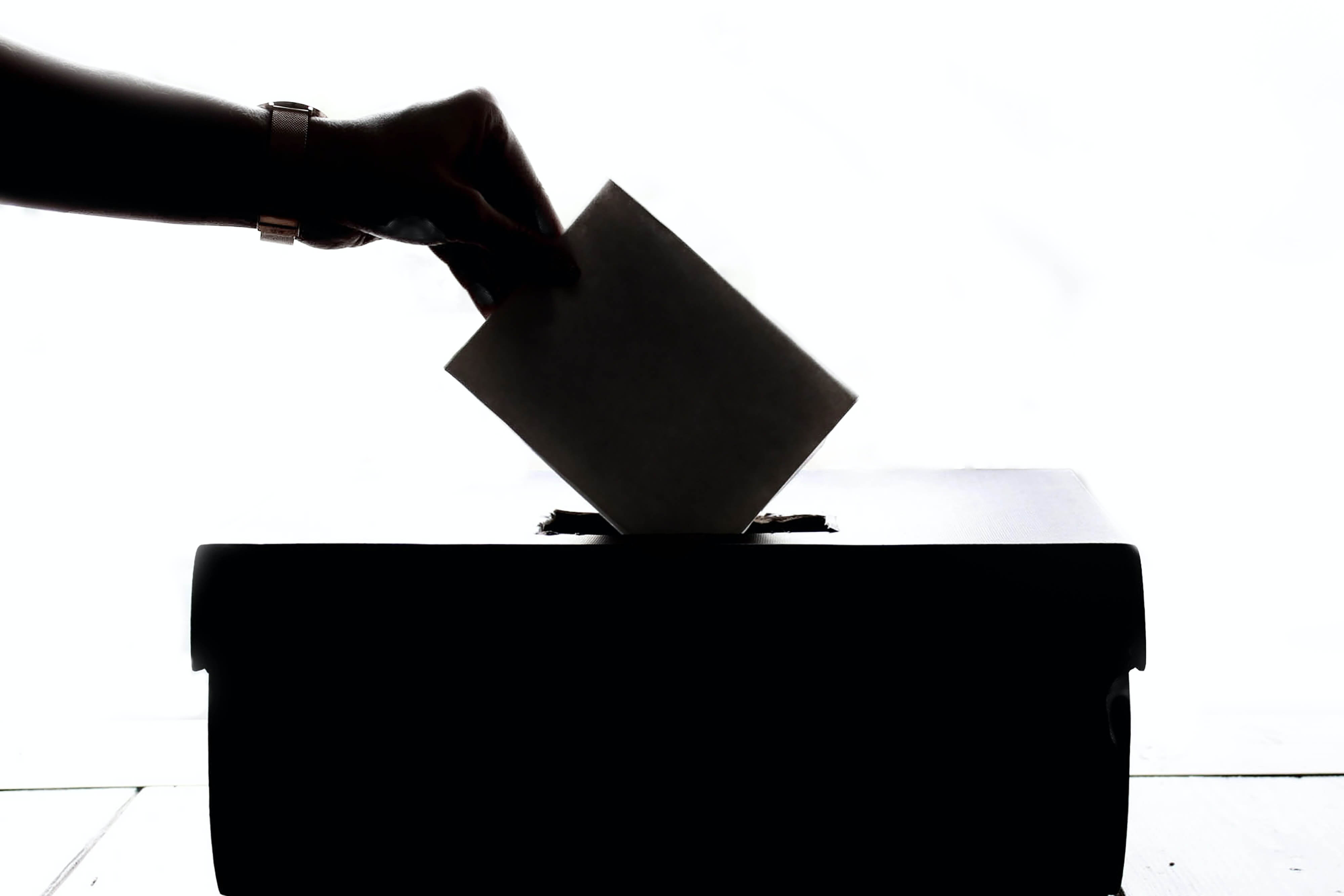
With the excitement surrounding opportunities that come with deploying Machine Learning (ML), it is easy to forget the downsides and risks. One of the major reasons that ML strategies lose money, miss the mark, or disappoint customers is ‘bad data’. Bad data can come in many forms and can lead to incorrect insights when improperly addressed and managed. Politics provide many examples of misuse of data and will be used to help illustrate the concept.
Before diving in, its useful to define a few common terms often used in data science. A population is defined as every member or every data point of a certain group. If we are hoping to understand voting intentions of Canadians in the upcoming election, we would say that all Canadians are included in the population. A sample, on the other hand, is a small number of datapoints that are drawn from the overall population. Think of a sample as 3,000 Canadian citizens who were contacted for a poll and asked their preferred candidate.
The first common issue when training ML models is simply not having enough data. When the sample size is small there can be bias and error introduced by chance. The data may contain outliers that have a large effect on the results because of their relative importance to the rest of the sample. Think of scrolling through twitter and trying to discern the political views of users. A single user with radical views can throw off your assessment and cause you to think these views represent a large part of the population.
Although it may seem intuitive that a small dataset may be misrepresentative, large datasets can fool managers and their machine learning algorithms as well. Think of how many polls are created and published during election time. It seems like every day there is a new poll proclaiming to know voter sentiment at that moment, only to find that they were way off as the true results come in.
A famous example comes from the U.S. election of 1936. Leading up to the election, a magazine called Literary Digest collected 2.4 million answers from readers and predicted the challenger, Alf Landon, would unseat President Franklin D. Roosevelt. FDR ended up taking the highest percentage of the popular vote since 1820. So what happened?
Managers need to think about the source of the data and the bias that may be introduced through the collection process. Readers of Literary Digest were upper class and were more likely to oppose the policies introduced by FDR. Also, those who tend to answer polls may have different opinions than those who do not answer polls. These differences do not show up in the data and lead to insights that do not represent the population.
Prior to training models, managers need to make sure they contemplate the data collection process, identify potential biases, and determine whether the dataset is truly representative of the overall population they are hoping to derive insights about. Keep in mind that data collection and analysis process is the job of data scientists. A manager's role is not to replace this expertise. Instead, the manager must ask critical questions about the data collection process and help identify potential sources of bias. Through this understanding, companies can avoid deploying models that contain harmful biases that affect the customers they hope to serve. This critical thinking will also help uncover the human biases present in organizations and may lead to constructive conversations on how machine learning can be created to benefit all.
Chapters
Trip Images
Want to stay in the loop?

Mitchell Johnstone
Director of Strategy
Mitch is a Strategic AI leader with 7+ years of transforming businesses through high-impact AI/ML projects. He combines deep technical acumen with business strategy, exemplified in roles spanning AI product management to entrepreneurial ventures. His portfolio includes proven success in driving product development, leading cross-functional teams, and navigating complex enterprise software landscapes.