The AI Hierarchy: crafting a successful AI strategy from the ground up
Part II: Crafting a Compelling AI Product Vision and Narrative
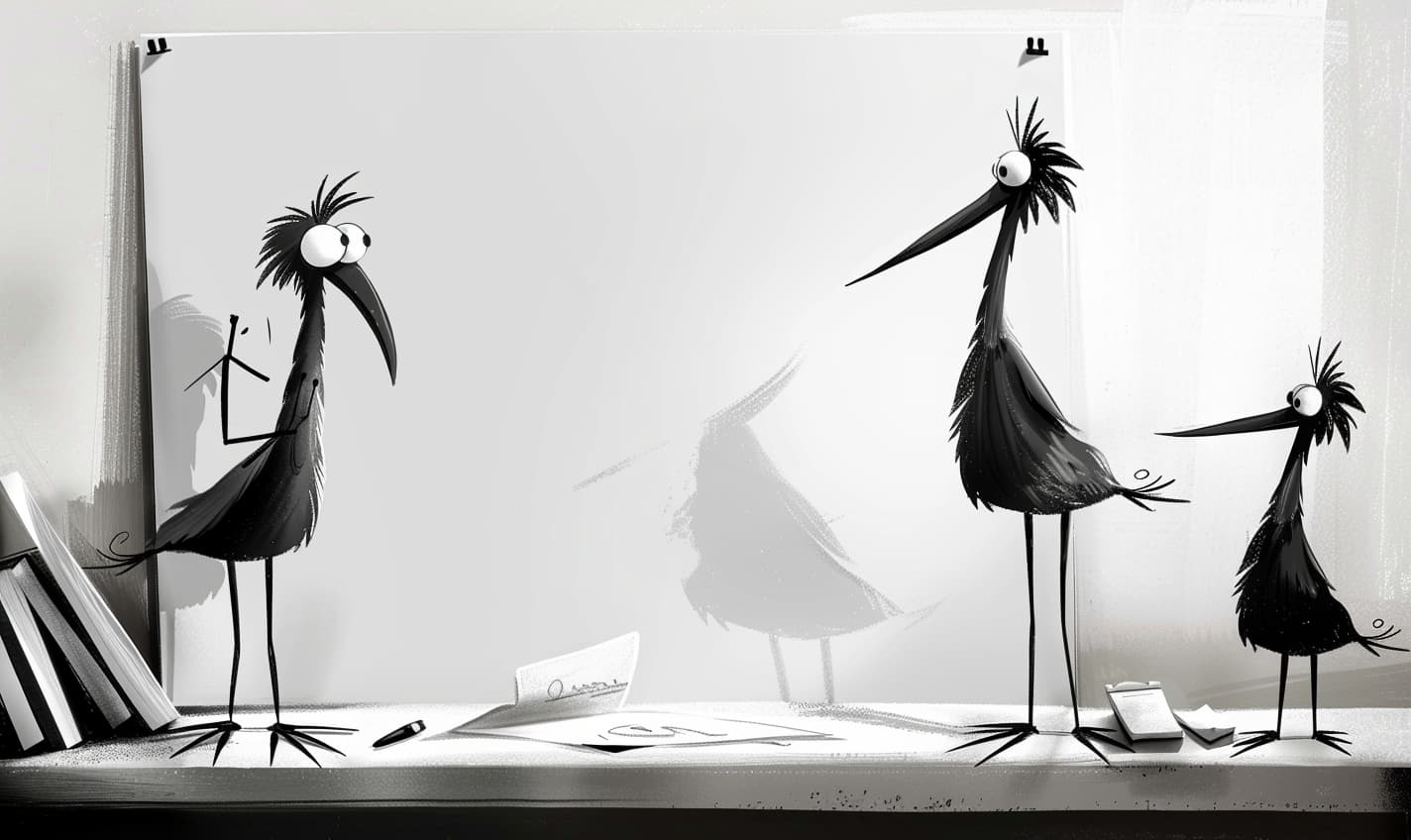
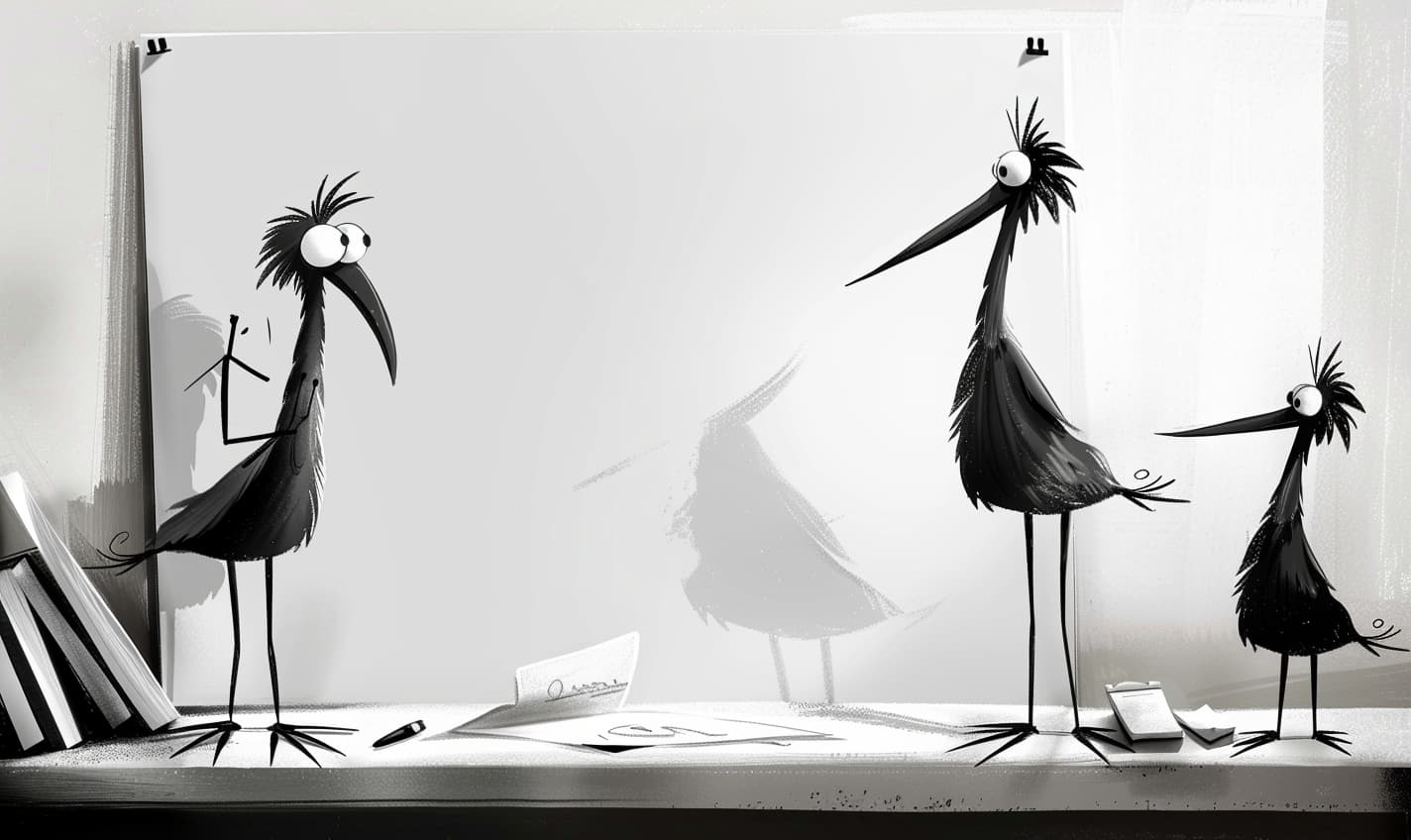
Itinerary
- Part I: Introducing the AI Strategy Framework
- Part II: Crafting a Compelling AI Product Vision and Narrative (👈You’re here)
- Part III: Data Collection - The Essence of AI
- Part IV: Ensuring Reliable and Accessible Storage
- Part V: Data Exploration and Transformation
- Part VI: Insights & Analysis - See the Unseen
- Part VII: Machine Learning - The Continuous Improvement Cycle
- Part VIII: AI & Deep Learning - Reaching the Pinnacle
The First AI Product Vision
Let’s first head back - way back. It’s the summer of 1956, and it’s the Dartmouth College Summer Conference. Our story begins with a gentleman by the name of John McCarthy. McCarthy was an exceptionally bright, young mathematician who, along with Marvin Minsky, Nathaniel Rochester, and Claude Shannon, were responsible for proposing the first AI workshop. McCarthy is also credited with coining the name ‘Artificial Intelligence’, and what is especially interesting for our purposes, is the proposal this group put together. Here is a brief excerpt:
“We propose that a 2-month, 10-man study of artificial intelligence be carried out during the summer of 1956 at Dartmouth College in Hanover, New Hampshire. The study is to proceed on the basis of the conjecture that every aspect of learning or any other feature of intelligence can in principle be so precisely described that a machine can be made to simulate it. An attempt will be made to find how to make machines use language, form abstractions and concepts, solve the kinds of problems now reserved for humans, and improve themselves. We think that a significant advance can be made in one or more of these problems if a carefully selected group of scientists work on it together for a summer.”
I love this proposal. It’s incredible how prescient and ambitious it was. Living now in the 21st century, we know that these gentlemen did not succeed in making ‘significant advances’ over the summer of 1956. In fact, we are the first to be living through what they proposed all those years ago. I know I’ve been blown away and captivated by the incredible powers of GPT-4 and other large language models widely available today. But this is not why I appreciate the proposal and why I bring this to your attention.
These men had a clear vision. They communicated it well and painted a picture of the future they wanted to build. This is the key foundational piece of every successful AI project. A vision, a narrative, a description of what could be in the future. Although these gentlemen lacked the tools and technologies that allowed that vision to become reality, we have those tools available today. To apply these tools and technologies successfully, we need to take the first page out of their proposal. We must first start with what we want to achieve.
The Amazon Approach
Though the Dartmouth group put together a single paragraph proposal, Amazon and other Silicon Valley companies have more formally applied this approach and tried to standardize the process of writing down and describing the future vision before executing. In their book Working Backwards, Colin Bryar and Bill Carr talk about Amazon’s approach to product management and how they use writing to more rigorously think about projects before they are formally proposed.
Here are the key points that Bryar and Carr make about the effectiveness of a written narrative vs. the typical powerpoint presentation:
- Greater Information Density: Narratives contain much more information than a typical PPT slide, allowing for a deeper dive into topics.
- Reading vs. Listening: People can read faster than they can listen, so narratives enable audiences to absorb more information in less time.
- Nonlinear, Interconnected Arguments: Narratives are better suited for complex, interconnected discussions that don't fit well into the linear format of PPT slides.
- Forces Clarity of Thought: Writing a narrative requires deeper thinking and anticipation of audience questions and objections. It helps in forming a more comprehensive argument.
- Bonus: Writing is even more accessible than before with the assistance of LLMs and can help those of different backgrounds and writing abilities to communicate their ideas clearly.
How do you write an Amazon-Style Narrative
There are many articles on the web that give examples and instructions on how to create an Amazon 6-pager, but even Bryar and Carr admit, there is no single way. Depending on the purpose and desired outcome, there can be many ways to structure an Amazon 6-pager. Here are their top tips:
- Six-Page Maximum: Aim for a functional length of about six pages to respect meeting times and maintain focus.
- Anticipate Questions and Objections: Address potential concerns and alternate viewpoints within the document.
- Clarity and Cohesion: Ensure the narrative is clear, persuasive, and logically structured. It should demonstrate how different points are interconnected.
How do we apply this to an AI Strategic Narrative?
The most important piece of the Amazon approach must be retained. Describe the problem, why it’s worth solving, and who has the problem. Start here - every time. Don’t worry about proceeding to the next section until you have enough clarity in your proposal that you could explain it to your grandmother.
Problem Validation
This section tells us why we are all here reading the narrative in the first place. There is a problem out in the world and we believe that we can solve it. To get everybody aligned and introduce them to the rest of the document, think about answering the follow questions:
- Who has this problem? Can we talk to them and ask them about it?
- Can we distil our research into easy to understand problem statements (eg. When taking action x, I want to do something I can’t yet do, so that I can achieve a goal.)
- Can we verify that this problem is something they would pay to have solved for them? How much would they pay?
Solution
Marty Cagan says that product teams spend way too much time on the problem space and not enough time on the solution space. The solution is where the magic is and if you get this wrong, you’ll never be successful. In this section you need to take a stand. Given the problem you’ve identified, what is your hypothesis? A good starting point is to use the following statement:
“We believe that by implementing this strategy and executing on our product vision, we will successfully move these key metrics in this specific direction.”
Things to avoid:
- Technical implementation details: this is a section where we describe what the solution is, not how it works. Let’s not get bogged down by technical details and ensure that a clear picture of our idea emerges and is digestible by a wide audience.
- Thinking small: later in the narrative you’ll take time to describe how you can incrementally get to the grand vision. In this section, however, it’s important to paint a picture of the fantastic future you imagine if the solution is built.
Target Outcomes
Layout the objectives
John Doerr, the famous Google investor, introduced Google's founders to the concept of OKRs early in the company's life. He explained them as a way to get organizational alignment and ensure that the company was all moving in the same direction. As part of your AI strategy you need to articulate the objectives - which is just a fancy way of saying the goals/outcomes you’d like to achieve. A good heuristic is 3 high level objectives and 3 key results (tangible metrics) that can be used to measure your progress against the goals you’ve set. Make sure that the goals are for no more than 6 months so that you can come back and re-evaluate consistently and modify if needed. Keep them clear, measurable, and ambitious.
Set a target
Which layer of the AI Strategy Framework Pyramid will this vision take us to? Does the use case end with data visuals? Is it a predictive program or recommendation software? Does it use Generative AI or Deep Learning to achieve our vision? By establishing the layer our vision takes us to, we can then work our way back down each layer of the pyramid and begin to detail the components we need to reach our target and fulfil our future vision. Make sure to mark which step your journey takes you to, whether it’s the data analytics, machine learning, or AI step. Then go back down to the bottom and begin uncovering how you will execute on data collection, and move step-by-step up the pyramid establishing tools, technologies, and strategy along each layer of the pyramid.
Minimum Viable Robot (MVR) - Delivering value at each step
No more thinking big, this section is where you get very granular and have a tangible plan to get to value as quickly as possible. The MVR - a play on the overused Minimum Viable Product acronym - is how we move fast. We need a way to deliver measurable value to the organization well before leadership gets tired of waiting for the promised vision to be realized and pulls the plug.
To do this, we can take a modified approach to working back up the pyramid in our AI Strategic Narrative document. Instead of focusing on the long-term vision, and all of the data, infrastructure, and data scientists we’ll need, we can instead focus on our quickest path to value. This smaller vision should not divert us from the path that leads to our bigger vision, we’re building the model airplane before we build the jet.
By focussing on a first smaller use-case, we get the benefit of a small target. Something that allows us to collect less data, introduce less complex technology, and lays the foundation for the future. We are taking a slice out of the AI Strategy Pyramid, building for the future while delivering value today.
Working Back Up - How we build the solution
Here is the section where some technical detail will be important. It is important to think through the tools, technologies, and processes that are going to allow you to reach your MVR target. Think your way through from the bottom up to your target.
Data Collection
Given the target you’ve set and the vision for the MVR, what data will you need to get there. By starting at the top and then moving back to the bottom, you should have a strong sense of what data will be required. If you are struggling, go back to the last step and get more specific about what you’re delivering.
Questions to think about:
- What types of data do we already have?
- How is this data collected (sensors, manual input, cookies, third party data)?
- How often is this data collected and what is our data size at each interval?
- Are there any real-time data streams?
- What file formats are we dealing with?
Data Storage
Next, you need a place to put the data that you’ve collected. Depending on the frequency, size, and file formats you’ll need to make a decision on the data storage location that is best for you. You may need many intermediate databases, or different fit-for-purpose databases and this is okay. Just make sure that you understand the purpose and function of each and how they fit into achieving your vision.
Questions to think about:
- Where is the collected data stored (cloud, on-premises servers)?
- What is the current data storage capacity?
- How is data security managed?
- Is there a system for data backup and recovery?
Data Transformation
Real world data is messy. It’s never in the format you need and often doesn’t have the associations to other data points you need to perform strong data analysis. Taking the raw data and cleaning up the values and structuring it in a way that data analysis can be performed is a key step on your journey.
Questions to think about:
- What processes are currently in place for cleaning and preprocessing the data?
- How is data normalized or standardized?
- Are there tools or software used for data transformation?
- How is the quality of transformed data ensured?
Data Analysis
Now you have a somewhat structured dataset with obvious errors cleaned up and a queryable structure. It’s time to dive in and see what the data has to say. Once again based on the data you expect to see, you can make decisions on how much compute and storage you will need to perform adequate data analysis. If you have a larger dataset you may select a different toolset than if you have a smaller data set.
Questions to think about:
- What tools or software are currently used for data analysis?
- Are there any automated processes for routine analysis?
- How are analysis results documented and reported?
- What challenges exist in the current data analysis processes?
Machine Learning
You’re almost there. The top of the pyramid is in sight. WIth a strong understanding of the data and a clean set ready for training you can begin experimenting with machine learning. Sometimes you’ll need different toolsets for analysis and machine learning so a decision will have to be made. More importantly, an engineering strategy for getting these models to work with the rest of your solution - MLOPs. This is often the most difficult part, so I highly recommend keeping the model simple and focus on getting the model out in the world.
Questions to think about:
- Are there existing machine learning models in use?
- How are these models trained and tested?
- What platforms are used for deploying these models?
- How is model performance monitored and evaluated?
- Are there processes for updating or retraining models?
AI & Deep Learning
You’ve done it! You’ve reached the top. Some of the concepts used in Machine Learning will be the same, you may be able to reuse much of your model infrastructure for deep learning, but also may need to make some key changes depending on your data volume and data types. Applying deep learning methods is more complex than machine learning so you may also need to hire. Training deep learning models can require more expensive hardware. Addressing all of these complexities from the start is daunting, no wonder this step is at the top.
Questions to consider.
- What specific deep learning applications are being considered?
- What is the current computational infrastructure available for deep learning (e.g., GPUs, cloud computing resources)?
- Are there datasets large and complex enough to warrant the use of deep learning?
- What expertise in deep learning exists within the team, and is additional training or hiring needed?
- How will the implementation of deep learning models integrate with existing systems and workflows?
Conclusion
Wrap it all up with a bow and get the team motivated to execute!
Example
Want to see an example? Leave us your email and we’ll send you a copy!
Wrapping Up
Think before you act. That is the essence of this step. Business plans get a bad rep, and maybe they should. But if you instead embrace Amazon's forward-thinking practices and the pioneering spirit of Dartmouth's early AI visionaries, you can propel your business into the future.
Next, in Part III, we'll dive deep into the essence of AI projects: data collection. We'll explore its pivotal role and introduce 'Data-centric AI'. See you next week!
Need Help?
If you're seeking to unlock the full potential of AI within your organization but need help, we’re here for you. Our AI strategies are a no-nonsense way to derive value from AI technology. Reach out. Together we can turn your AI vision into reality.
Chapters

Mitchell Johnstone
Director of Strategy
Mitch is a Strategic AI leader with 7+ years of transforming businesses through high-impact AI/ML projects. He combines deep technical acumen with business strategy, exemplified in roles spanning AI product management to entrepreneurial ventures. His portfolio includes proven success in driving product development, leading cross-functional teams, and navigating complex enterprise software landscapes.